
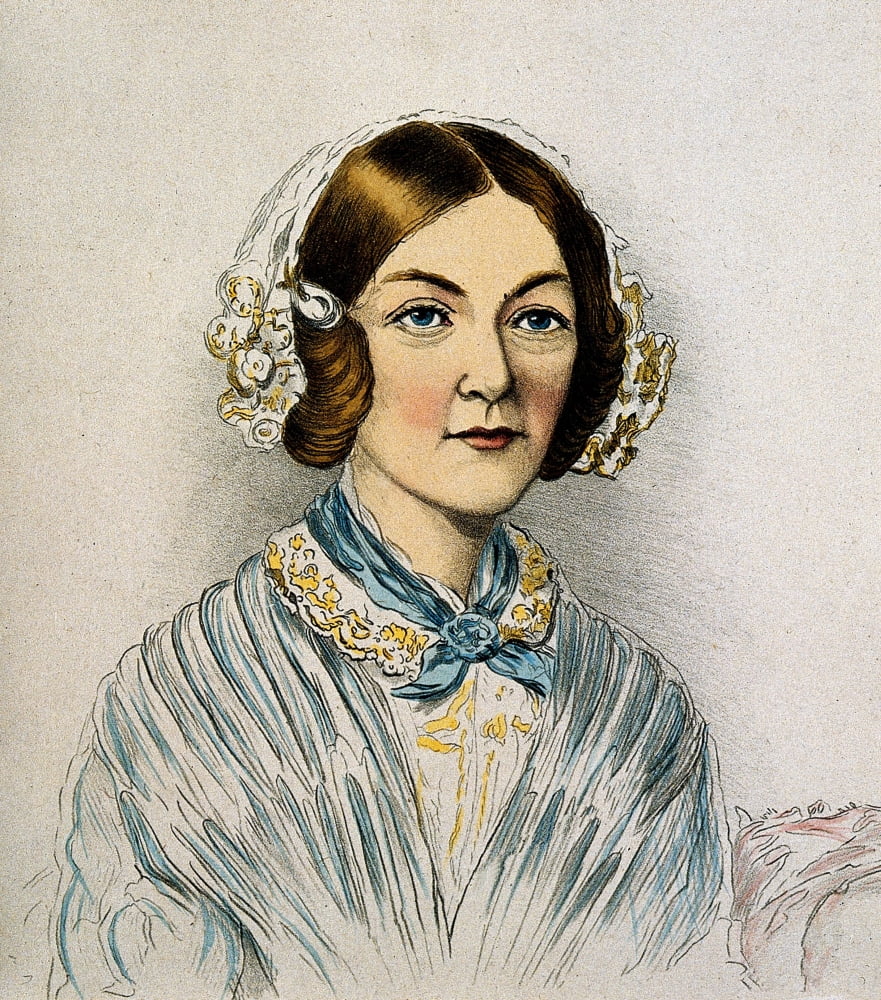
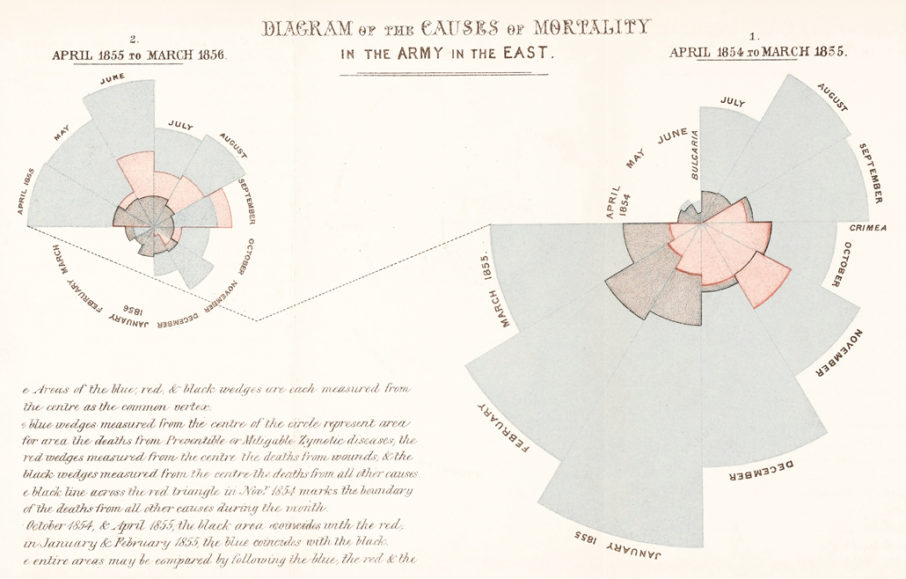
Technologies that allow computers and machines to function in an intelligent manner, often referred to as artificial intelligence (AI), are now being applied in citizen science. Members of the public are making substantial contributions to science as citizen scientists, and advances in technologies have enabled citizens to make even more substantial contributions. An appraisal of recent literature was also presented and knowledge gaps and future perspective in the research area were proposed. Biochemical oxygen demand (BOD) was the most investigated parameter in surface water quality monitoring and assessment. There was no clear relationship between data size and R 2 value (at the testing stage). ANFIS, Wavelet-ANN (W-ANN) and Wavelet-ANFIS (W-ANFIS) were most accurate for the prediction of surface water quality. Most of the studies using Neural Networks in surface water quality monitoring and assessment are originated in Iran and southeast Asia. From the study, it was observed that Adaptive Neuro-Fuzzy Inference System (ANFIS) and Artiicial Neural Networks (ANN) are the most uti-lised artiicial intelligence models for water quality monitoring and assessment in the last decade. Furthermore, the main contribution of this paper is to synthesise the existing body of knowledge in the state of the art (the last decade) and identiied common threads and gaps that would open up new challenging, exciting and signiicant research directions. The analysis focused on the methods used, the location of the experiments, the input parameters, and the output metrics applied to categorise the results presented. The goal of this paper was to conduct a systematic literature analysis on the application of diferent types of artiicial intelligence models in surface water quality monitoring. The approach was tested by simulated datasets collected from multiple marine data sharing websites, and the result suggested that the proposed method exhibits better effectiveness. Then, data correlation was calculated based on the literature on marine science data and ranking of the recommendation list to obtain the re-rank recommendation list. Data similarity was calculated by analyzing the subject, source, spatial, and temporal attributes to obtain the recommendation list.
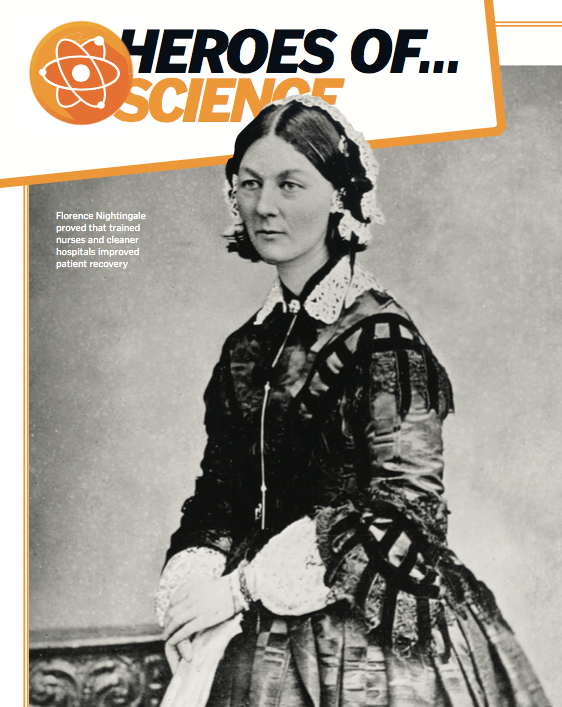
In this study, an approach was proposed based on data similarity and data correlation. The two most popular recommendation algorithms are collaborative filtering algorithms and content-based filtering algorithms, which may not work well for marine science observation data given the complexity of data attributes and lack of user information. A recommendation system is an effective method to improve retrieval capabilities to help users obtain valuable data. With the development of ocean exploration technology and the rapid growth in the amount of marine science observation data, people are faced with a great challenge to identify valuable data from the massive ocean observation data.
